Deep Learning for Mortgage Risk
Kay Giesecke
Founder, Chairman and Chief Scientist, Professor at Stanford University
Justin Sirignano
University of Oxford
Apaar Sadhwani
Google Health AI
This paper examines the behavior of mortgage borrowers over several economic cycles using an unprecedented dataset of origination and monthly performance records for over 120 million mortgages originated across the US between 1995 and 2014. Our deep learning model of multi-period mortgage delinquency, foreclosure, and prepayment risk uncovers the highly nonlinear influence on borrower behavior of an exceptionally broad range of loan-specific and macroeconomic variables down to the zip-code level. In particular, most variables strongly interact. Prepayments involve the greatest nonlinear effects among all events. We demonstrate the significant implications of the nonlinearities for risk management, investment management, and mortgage-backed securities.
This paper is published in the Journal of Financial Econometrics, volume 19(2), pages 313–368, 2021.
Request Download
About the Speaker
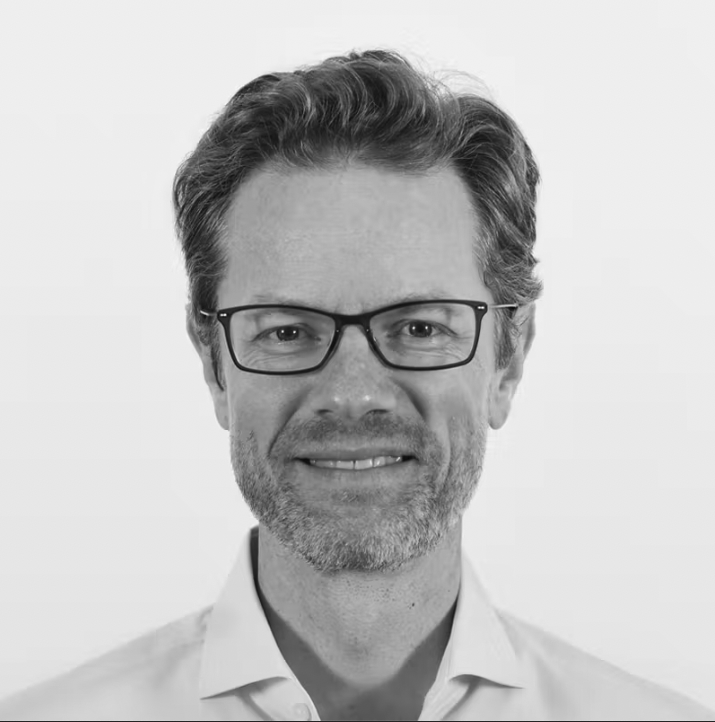
Kay Giesecke
Founder, Chairman and Chief Scientist, Professor at Stanford University
Kay Giesecke is the Founder, Chairman and Chief Scientist at Infima. He is also Professor of Management Science & Engineering at Stanford University, the director of the Advanced Financial Technologies Laboratory, and the director of the Mathematical and Computational Finance Program. Kay serves on the Governing Board and Scientific Advisory Board of the Consortium for Data Analytics in Risk. He is a member of the Council of the Bachelier Finance Society.
Kay is a financial technologist interested in solving the challenging modeling, statistical, and computational problems arising in fixed-income and credit markets. Together with his students at Stanford, Kay has pioneered the core elements of the deep learning and computational technologies underpinning Infima’s solutions.
Kay’s research has won several awards, including the JP Morgan AI Faculty Research Award (2019) and the Fama/DFA Prize (2011), and has been funded by the National Science Foundation, JP Morgan, State Street, Morgan Stanley, Swiss Re, American Express, Moody's,and several other organizations.
Kay has advised several financial technology startups and has been a consultant to banks,investment and risk management firms, governmental agencies, and supranational organizations.
Justin Sirignano
University of Oxford
Justin is an Associate Professor of Mathematics at the University of Oxford and Director of the Oxford Masters program in Mathematical & Computational Finance. Justin's research lies at the intersection of applied mathematics, machine learning, and high-performance computing and is focused on theory and applications of Deep Learning. Justin develops deep learning models for large financial datasets such as: high-frequency data from limit order books, loans, and options. He is also developing deep learning methods for constructing partial differential equation models from data, which has a variety of applications in science and engineering. Justin received his PhD from Stanford University and holds a Bachelors degree from Princeton University. He was a Chapman Fellow at the Department of Mathematics at Imperial College. He was awarded the 2014 SIAM Financial Mathematics and Engineering Conference Paper Prize.
Apaar Sadhwani
Google Health AI
Apaar Sadhwani started his career as a Research Scientist at Google Brain. He currently is a Technical Lead at Google Health AI. He obtained a PhD in Management Science and Engineering at Stanford University and a MS in Mathematical and Computational Finance, also from Stanford. In his PhD research, Apaar developed and analyzed deep learning models of mortgage borrower behavior and designed online authentication policies for the world's largest biometric system. Apaar has a proven track record in product-driven research with his work being covered by several media outlets. He is enthusiastic about solving interdisciplinary problems involving both technical and cross-functional challenges.